Foundational Level Course
Statistics for Data Science I
The students will be introduced to large datasets. Using this data, the students will be introduced to various insights one can glean from the data. Basic concepts of probability also will be introduced during the course leading to a discussion on Random variables.
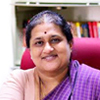
by Usha Mohan
Course ID: BSMA1002
Course Credits: 4
Course Type: Foundational
Pre-requisites: None