Degree Level Course
Introduction to Natural Language Processing (i-NLP)
Natural language (NL) refers to the language spoken/written by humans. NL is the primary mode of communication for humans. With the growth of the world wide web, data in the form of text has grown exponentially. It calls for the development of algorithms and techniques for processing natural language for the automation and development of intelligent machines: Natural Language Processing (NLP). On the completing the course, the participant will learn the following: 1. Why is processing language computationally hard and why specialized techniques need to be developed to process texts? 2. Knowledge and in-depth understanding of linguistics techniques and classical (statistical) approaches (pre-deep learning era) to NLP and their limitations. 3. Knowledge and in-depth understanding of deep learning approaches (RNN and CNN) to NLP. 4. Knowledge and in-depth understanding of Attention Mechanism, Transformers and Large Language Models (LLMs) 5. Ability to read and understand latest NLP-related research papers. 6. Ability to identify applicable NLP technique to solve a real-world problem involving text processing. 7. Ability to implement NLP models and algorithms for problems related to text processing. 8. Ability to develop applications based on textual generative models (LLMs - Large Language Models)
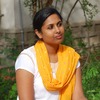
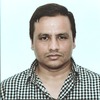
Course ID: BSCS5002
Course Credits: 4
Course Type: Elective
Pre-requisites: BSCS3004 - Deep Learning