Diploma Level Course
Machine Learning Techniques
To introduce the main methods and models used in machine learning problems of regression, classification and clustering. To study the properties of these models and methods and learn about their suitability for different problems.
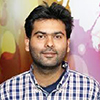
Course ID: BSCS2007
Course Credits: 4
Course Type: Data Science
Pre-requisites: None
Co-requisites: BSCS2004 - Machine Learning Foundations